A Prometheus Moment: Artificial intelligence in healthcare’s evolution
As the excitement around AI explodes, here’s where it stands at crossroads of innovation, ethics and expectations in AI’s healthcare journey.
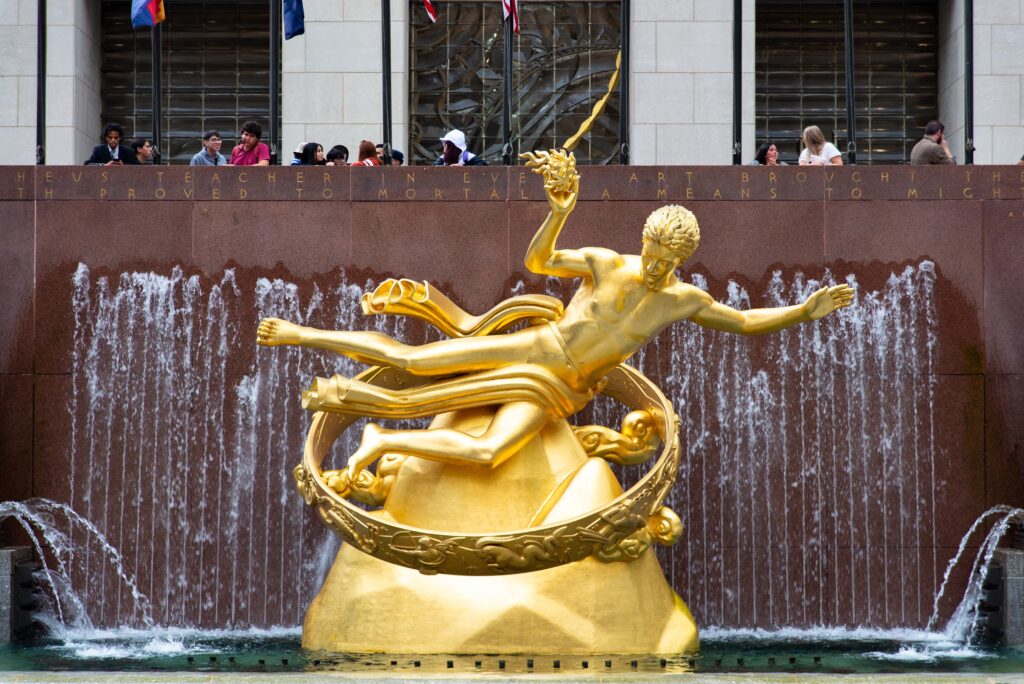
Artificial intelligence – the Hype Cycle meets Fright Night.
The cluster of technologies that represents this advanced technology lies in a strange vortex right now. Expectations have been high, in some cases stratospheric. Swirling in the mix is a range of high-profile concerns, fear and panic about the unbridled use of AI.
At the same time, there’s great confusion about these technologies, the various flavors of which tend to merge in the minds of many. And despite the vagaries of all these factors, hopes for AI to make a profound impact in healthcare delivery have never been higher. It’s time for technology to deliver to automate documentation, winnow down the information that clinicians see, reduce clinicians’ burden, and provide an extra set of knowledge to improve patient outcomes.
In spite of the perils, AI holds that much promise, contends John Halamka, MD, president of the Mayo Clinic Platform. “It’s an extraordinary time … for health data management,” he says. “For those of us who have been in the industry for multiple decades, I think we’re seeing a turning point for healthcare. So, I’m calling this the Prometheus moment,” a sense that new tools, ways of thinking and capabilities will offer a radical departure that will extensively alter many aspects of the industry.
Fears allayed by its potential
The soaring expectations come against common confusion about what artificial intelligence is, how far along certain capabilities are in maturity and how easily it can fit into common healthcare practice.
AI is an “umbrella term for a lot of subsets of science,” explained Elliott Stepusin, director of machine learning engineering for Imprivata, during a recent Illinois Chapter meeting of HIMSS. Machine learning, natural language processing and large language models are smaller components of AI that feed the capacity for AI to learn from experience or data, adjust to new inputs and perform tasks.
AI’s predictive capabilities have been around for a while – feeding suggested videos to you on Netflix or YouTube, or suggesting possible purchases on merchandise websites. Since the middle of the last decade, there’s been increased excitement – and concern – about AI’s ability to bring predictive and diagnostic capabilities to healthcare, and expectations have really soared with the popular around generative AI technologies, such as ChatGPT, which can create human-understandable content and mimic human creative thought processes.
The rollout of AI in healthcare has not always been managed well, evoking fears among some clinicians that it may eventually replace human clinical decision-making with mysterious, unchallenged algorithms fed with inconclusive or biased data.
“AI does create a sense of concern or panic in folks,” affirms Shruti Mehta Cruz, director of digital solutions for information services at Northwestern Medicine, a Chicago metro healthcare system. “You have to counteract the idea that it is all-knowing and can do everything that a person can do. In our terminology, we’ve pivoted to (calling it) augmented intelligence, because we do want to keep humans in the loop – it enhances instead of replacing humans.”
High hopes, but it’s early
It's not all fear among clinicians, who are increasingly appreciating what AI can do to help them. For example, an American Medical Association survey of 1,081 physicians found that respondents are “enthusiastic about augmented intelligence ... and its assistive role in healthcare that enhances human intelligence rather than replaces it.” Data suggests respondents are most excited about AI tools that help reduce administrative burdens, including documentation (mentioned by 54 percent of respondents) and prior authorization (48 percent).
“The AMA survey illustrates that physicians’ greatest hope for AI rests in reducing the crushing administrative burdens that plague modern medicine, which drain healthcare resources and pull physicians away from patient care,” said AMA president Jesse M. Ehrenfeld, MD.
Early applications of some AI-infused technologies have shown promise in reducing clinician burden. For example, the American Academy of Family Physicians surveyed members on their use of Navina, an AI-powered platform that offers an assistant that uses generative AI to support doctors’ clinical and administrative tasks. Respondents said the technology helped reduce preparation time by 38 percent, improved perceptions of clinical care performance and practice satisfaction, while easing burnout pressures.
Still, implementation of AI is not widespread. The 2024 Industry Outlook survey from eClinical Solutions found that 80 percent of organizations it surveyed said they are in the exploratory stage or are planning to implement AI or machine learning. By contrast, only 7 percent say they have integrated AI or ML across one or multiple applications. Even so, hope remains high, with 53 percent of respondents indicating that AI and ML are their top trends to watch this year, compared with 11 percent last year.
And despite the hype around generative AI, some adoption is occurring, according to a recent analysis by KLAS Research. In a report issued in December, the HIT research organization noted that “the number of organizations adopting generative AI solutions is relatively small, and most who have adopted say it is too early to report outcomes.” Those systems that have tried generative AI solutions tend to be larger, which tend to have more resources, more data and the capacity to hire data scientists and specialists who can help drive outcomes from solutions.
Still, the buzz around AI is permeating healthcare organizations, says Cruz of Northwestern Medicine. “AI has dominated a lot of conversations at the board level, and we try to contain expectations,” she says. “It’s important to go back to the basics – what is the problem we’re trying to solve, rather than just looking at a shiny solution. We’re thinking about AI as another tool within our toolkit. Some problems can be solved by process improvement, and we’re seeing if AI can help fill in gaps. We try to get out of the concept that AI can just solve any problem – do we even have the data to model and solve this problem?”
Understanding the differences
Part of the divergence in expectations is what is possible and real at this moment, and what is developmental yet overhyped at this time. Mayo’s Halamka notes this bifurcation in describing the expectations surrounding predictive AI and generative AI.
“We know a lot about predictive AI – I’ve been working on various kinds of (predictive AI) models since the 1990s,” he says. “Generative AI is an entirely different animal, because it’s very hard to assess the accuracy and the quality. There’s no transparency about how it is trained. And if we’re going to have credible generative AI, it needs to have these characteristics.”
There’s growing efforts to test predictive AI solutions. At Mayo, the organization has tested 242 finished AI models, Halamka says. Several have gone through validation by the Food and Drug Administration and other oversight agencies, and he estimates that about 20 percent are actually in use in day-to-day practice.
Mayo and other organizations hope to expand the use of validation efforts for AI. They’ve recently announced the formation of a nonprofit joint venture that’s a partnership of academic hospitals and technology companies with the goal of establishing a network of laboratories for testing AI tools intended for use in healthcare, with the hope of certifying and registering them. The initiative, to be built out this year, includes Mayo, Duke Health, Stanford Health Care and other large universities and organizations.
A broader initiative was announced in January, as the U.S. National Science Foundation and other agencies launched the National Artificial Intelligence Research Resource pilot, described as a first step toward a shared research infrastructure intended to strengthen and democratize access to resources that can support responsible AI discovery and innovation. The initiative is backed by 10 other federal agencies and 25 private sector, nonprofit and philanthropic organizations, including the Regenstrief Institute.
Other healthcare provider organizations are implementing AI predictive models where it makes sense, looking to achieve demonstrable benefits.
For example, UC San Diego Health is using a sepsis prediction model, which has demonstrated “improvements in its sepsis bundle compliance,” says Karandeep Singh, MD, recently named as its chief health AI officer. The organization “has done simulation studies and showed that they’ve potentially save a bunch of money as a result of giving better sepsis care.” Singh says the organization also uses predictive AI to estimate censuses at its facilities.
At Northwestern Medicine, a couple AI solutions are being implemented, Cruz notes. One focuses on measures to improve patient safety and risk management, while another looks through imaging reports to locate incidental findings that require follow-up, integrating them into a workflow in its Epic records system so such findings in reports are linked to appropriate follow-up care. The guiding principle is “where there is true value, does it help to mitigate safety risk or can it achieve savings,” she adds.
Generative AI
Use of generative AI in healthcare is in the early stages, in part because of the newness of applications and also because of questions surrounding how it can be safely used.
Its capabilities have been worked on for years, Halamka notes. “This is still a journey for us – generative AI is a revolution 50 years in the making. We couldn’t have gotten to this place unless we spent decades on data standards and normalization and interoperability, and then started building cloud structures with privacy protections, and compliance oversight and auditing.
“With generative AI, you have to be a little more careful with the use cases … and the risks you’re willing to tolerate,” he adds.
In contrast to esoteric and untested uses for generative AI, organizations are looking to use the technology to relieve clinicians of documentation and coding burdens, lightening the administrative load, and potentially facilitating some currently human-heavy tasks that can potentially be automated.
Some of that capability is already in place, when generative AI is paired with ambient listening technologies, which can help clinicians draft encounter notes by listening to conversations between physicians and patients and writing a first draft of the physician’s note for the electronic record. In January, Epic announced DAX Copilot integrated into its EHR system, which it says will enable more than 150 health systems, hospitals and medical centers to automate clinical documentation. The integration is the result of a partnership between Microsoft, Nuance and Epic.
Singh says UC San Diego Health is one of the first health centers in the country to use generative AI and large language models to communicate with patients. “I think there’s a wave of interest there,” he says. “I think we have something like 15 generative AI pilots currently in progress and just starting to launch.”
Still, the worries remain
Still, several issues surround the use of generative AI, including data security and privacy, data inputs and large language model capabilities, ongoing testing and improvement of algorithms, and oversight of technologies, particularly as patient care uses are contemplated.
Initiatives and guidelines from the U.S. government and World Health Organization seek to address some of these concerns, aiming to intercept some of the fears that have arisen over unbridled use of the technology by healthcare and other industries.
The Coalition for Health AI is formalizing its approach to oversee the use of the technology in healthcare. Last year, the organization unveiled a blueprint for the use of trustworthy AI in healthcare, and now 1,300 companies are participating in the initiative, Halamka says. It’s formalized its governing structure, activities and workgroups, hoping to develop a testing process to certify AI products and keeping a registry of approved applications, similar to the Certified Health IT Products (CHPL) list of certified EHR technology during the Meaningful Use program.
AI products that impact clinical care need other oversight, either from clinical organizations or government agencies, to alleviate concerns and oversee efficacy and safety.
In any event, AI is likely to play an important role in healthcare as the world deals with demographic, financial and ethical challenges facing healthcare organizations in the years ahead.
In attending the recent World Economic Forum in Davos, Switzerland, Halamka found worldwide concern that leaders are worried about a growing workforce supply-demand mismatch – where an aging population will need more care even as the healthcare workforce shrinks.
“The thinking was, ‘Let’s address some of the societal demographic shifts by asking what work can be done by predictive and generative AI when we have just too few people to do the work that needs to be done,’ “ he says. Much work lies ahead, he emphasizes. “The challenge, of course, is that AI is trusted by many people – the technology is so good, it’s indistinguishable from magic. And so we have to be a little careful that you don’t just trust AI because the AI seems magical.”