Navigating the data highway: Lessons from the seat belt
How harnessing high-quality data can pave the way for advanced healthcare solutions and better patient outcomes.
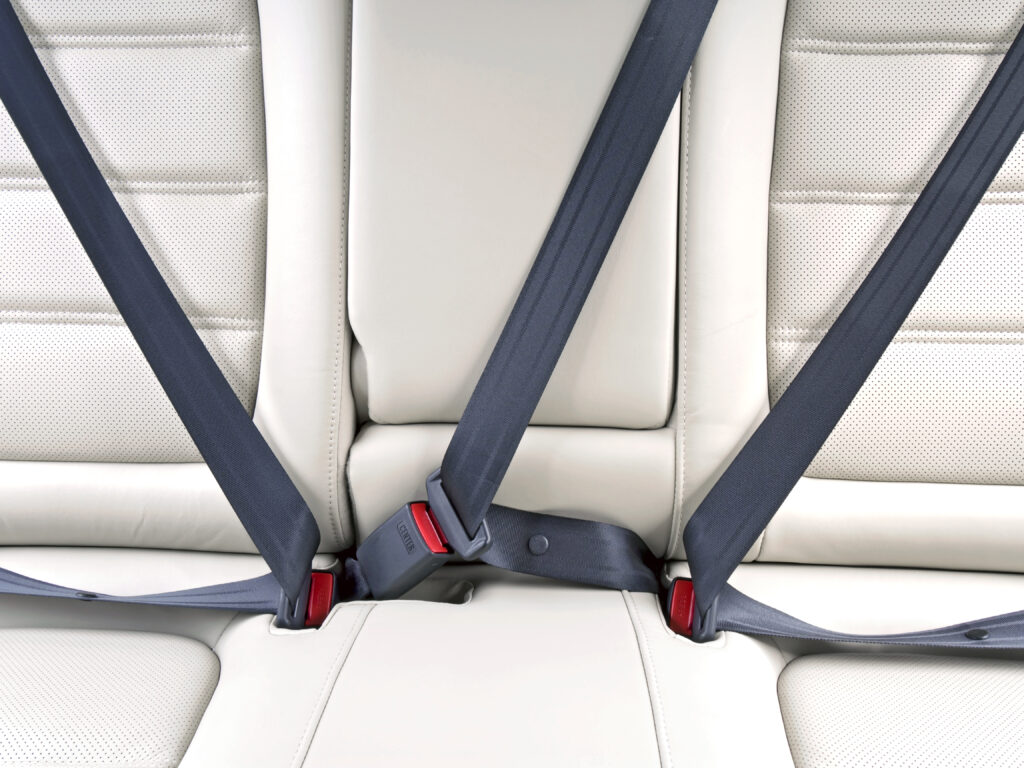
The road to transformation in healthcare is paved with the thoughtful use of data. The challenges and opportunities, much like the amount of data itself, grow daily. Here, I’ll discuss how it’s possible to overcome them with the adaptation of an essential component of passenger safety –the seat belt.
Consider this – the healthcare industry generates approximately 30 percent of the world's data volume, with the compound annual growth rate of healthcare data forecasted to reach 36 percent by 2025 (The Digitization of the World — From Edge to Core, IDC White Paper, 2018). Consequently, data platforms and analytics tools are quickly becoming table stakes to succeed — but how can users leverage them for maximum impact?
To understand how organizations can thrive in this evolving environment, Arcadia recently partnered with the Healthcare Information and Management Systems Society (HIMSS) to conduct a pulse survey on the current state of healthcare analytics platforms. The findings, The Current State of Healthcare Analytics Platforms, revealed that organizations understand the value of data but struggle to use it effectively. Data quality and trust, which go hand in hand, are among the most common pitfalls — but there are strategies healthcare organizations can deploy to surmount them.
Technological and data challenges
Most data challenges within healthcare can be attributed to accuracy. Some 22 percent of healthcare leaders cite inaccuracy of data as a challenge their organization faces, and nearly a third say their data is less than 76 percent accurate, according to the HIMSS and Arcadia research.
There are three main pitfalls that can render data inaccurate and consequently reduce audience trust in the analytics derived from it.
Data completeness. Failing to curate or aggregate all the necessary data for input sources can leave organizations with a hazy, incomplete picture of the landscape. This could result from missing entire sources of data within a siloed ecosystem or simply from not taking full advantage of the data that is present — often by failing to tap into unstructured or pseudo-structured elements.
Data transformation. An analytics program’s output is only as good as its input, but it is also only as good as the transformation that the data undergoes to be fit for its intended purpose. The accuracy of data interpretation (for example, was this blood pressure reading from a sitting or supine position?) as well as data computation (for example, what was the final cost of this claim after all adjustments are accounted for?) are major contributors to the accuracy of any analytic output.
Data timeliness. Some 20 percent of healthcare leaders say delayed response times or the inability to produce data in real-time is a challenge they face. Data platforms should be highly liquid and updated often with fresh data easily surfaced so the end user receives a timely snapshot, according to the HIMSS and Arcadia research.
Improving healthcare’s future
One strategy in the face of these woes is a concept I call data seat belts, a series of safeguards that ensure high-quality, trustworthy data over time. It’s a tried-and-true component of passenger safety adapted to the science of healthcare data.
Think of data networks like roadways – each one of us is responsible for piloting our own vehicle safely and responsibly, but other drivers or even inclement weather could cause an accident, regardless of your thoroughness.
Organizations can approach data management by adapting essential safety measures introduced to passenger vehicles seven decades ago – seat belts. So-called “data seat belts” can minimize risk and ensure data quality, so that if you end up in a data crash, your audience is protected from harm and can continue using and trusting their data.
In practice, data seat belts are a strong portfolio of checkpoints for advanced analytics that could include:
Incremental dataset checks. Healthcare data is constantly changing. Users and administrators must ask themselves, for each batch, did I get the data I expected? Was it in the right format? Did the business information it contained align with previous batches? For EHR data, this could look like measuring the volume over time of key clinical concepts. For claims data, this could look like comparing the member months and per member per month costs for each month against the same values from the previous data load.
Quality measure checks and other batch analyses. Frequently, upstream data issues can introduce major inaccuracies in downstream results, such as quality measures used to evaluate performance in value-based care. Users and administrators must ask themselves, what was the percent change in the denominator and the numerator of my quality measure compared with the last calculation? Is that change within the expected range for that measure?
Action trigger checks. For suggested actions informed by data to be trusted at the point of care, they must be reliable. Users and administrators must ask themselves, are the clinical actions my data is triggering consistent with previous information both in volume and content? Is there a big spike that could indicate an upstream data issue or a slow drift over time that could indicate a more silent issue?
A substantial benefit of good data management is that it builds trust in both the data itself and the advanced analytics that are produced as a result. The best ongoing practices assume that something will go wrong, flag it when it does, and isolate that data from moving downstream and impacting users.
Leveraging data seat belts
Digital transformation and payment reform are radically changing the healthcare industry, and with these changes come new pressures and challenges. To succeed, there’s an acute need for healthcare organizations to lean into technology to improve the way they manage and deliver care.
To navigate these changes and achieve successful transformation, healthcare organizations must use the advanced approaches and tools that streamline the entire data lifecycle, from collection to storage to sharing to analysis.
Among those who use such tools, satisfaction is high. Some 82 percent of healthcare leaders are satisfied with their current analytics platform, according to the HIMSS and Arcadia Market research. So are the strong financial results these organizations deliver – those using a data platform outperformed the Medicare Shared Savings market in savings and bonuses alike, achieving 9 percent more savings and 22 percent more in bonuses. In 2020, Arcadia’s MSSP ACO customers generated $386 million in shared savings, averaging $10.7 million each, according to a September 2021 press release.
Now more than ever, data is the currency that will enable healthcare organizations to deliver high-quality care and do it efficiently, as well as generate financial results to thrive in an evolving economic climate.
Successful organizations also will deliver on modern patient expectations and improved health outcomes, enabling happier, healthier days for the populations they serve.
Mary Kuchenbrod is vice president of data operations for Arcadia.