How insurers can use data analytics to make better decisions
Insurance companies are looking to use artificial intelligence and machine learning to optimize efficiency and reduce costs.
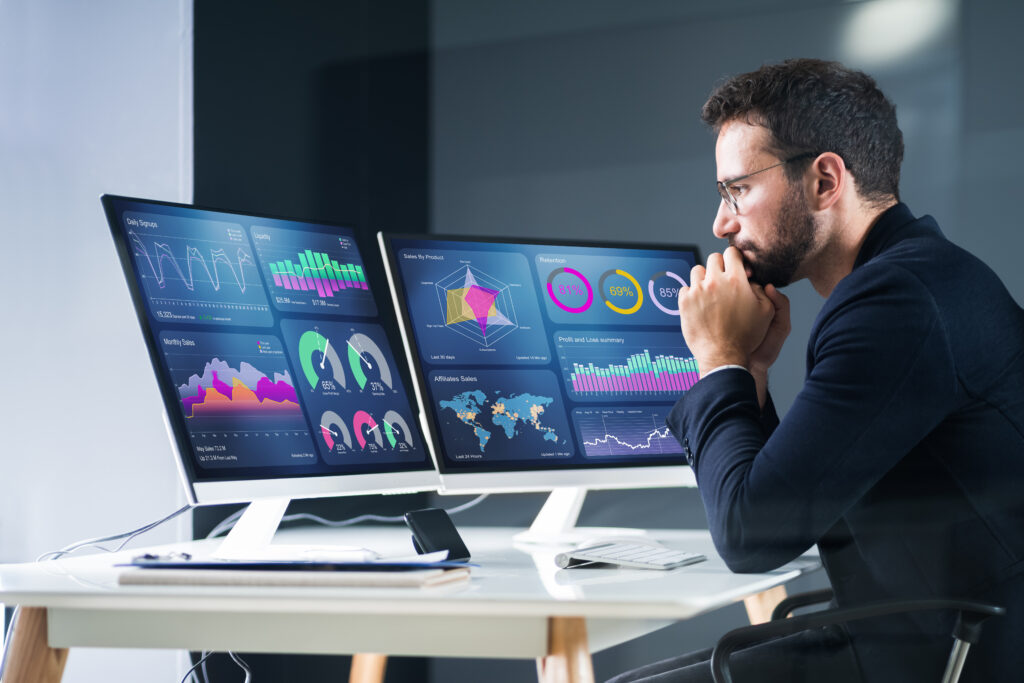
In the healthcare insurance industry, data analytics — the process of extracting useful information from raw data — plays an increasingly critical role in helping insurers improve efficiency and reduce costs.
Descriptive analytics uses present and past data to understand an organization’s conditions and operations, while predictive analytics deploys the same data to forecast future trends and developments. The insights gained from data analytics can be used to automate tasks, identify fraud and prevent waste.
The shift from batch-based to real-time data collection and analysis is transforming the health insurance industry. Insurers of all sizes are using data models and predictive analytics to better understand their members’ needs, develop new products and services, target interventions, and improve preventive care. Harnessing the power of big data and predictive analytics can reduce costs and improve outcomes for insurers and members.
Uses of data analytics in healthcare
The day-to-day operations of the healthcare insurance industry generate an ever-increasing amount of data that can be analyzed and leveraged. This includes patient data, such as demographics, income, health history, insurance claims and records of costs paid by all parties. Risk-scoring algorithms calculate the healthcare claims costs an individual is likely to incur relative to the average.
Additionally, predictive analytics can identify members at high risk for developing health problems, including chronic diseases and suggest additional screenings or lifestyle adjustments, resulting in improved health outcomes for the member and reduced costs for the insurer.
Models that use real-time data can continuously be updated with details about claims and benefit utilization and provide members with the latest advice. For example, users can ask a model whether they are eligible for a service and instantly receive the answer.
Real-time data analysis also can improve utilization management, which involves evaluating the necessity of a healthcare service before it is administered to reduce unnecessary costs. Additionally, data analysis can identify user behavior patterns and identify fraudulent claims.
Data collection facilitates the automation of certain parts of the healthcare insurance workflow. Automating healthcare transactions, including benefit verification, referrals and claim inquiries, has saved the healthcare industry $187 billion annually. This became especially important as the number of administrative transactions in the industry increased after the COVID-19 pandemic. Reports and visualizations that summarize important data for stakeholders also can be automatically generated. Having easy access to clear visual overviews of data can provide insights that lead to better, more informed decision-making.
Many healthcare providers are already reaping the benefits of data analytics. For example, integrated healthcare consortium Kaiser Permanente has implemented a data exchange system that saved them about $1 billion.
Similarly, the Centers for Medicaid & Medicare services have prevented $210 million in fraud-related losses using predictive analytics. As analytical tools become more sophisticated, the possibilities for cost savings will also continue to grow.
Tools for analyzing healthcare data
Because data generated by the healthcare insurance industry comes from a variety of sources and is typically unstructured, successful analysis must be able to integrate a large amount of complex, heterogeneous data. Mining, visualizing and interpreting the data are just as important — if not more so — as collecting it.
Examples of data mining techniques useful in healthcare include anomaly detection, clustering and classification. Artificial intelligence (AI) and machine learning (ML) are increasingly being employed in healthcare analytics.
Healthcare data analytics typically utilizes high-level programming languages such as R, Python and Tableau. Software solutions for benefit administration are commercially available and widely used by public and private insurers, including UnitedHealth, Aetna and CMS.
Challenges related to healthcare analytics
While there are numerous benefits to the use of healthcare data analytics, collecting user data raises data compliance and security issues.
The Health Insurance Portability and Accountability Act of 1996 (HIPAA) and other insurance guidelines regulate individuals’ healthcare data use. As such, it is vital for organizations to prioritize compliance and customer privacy, and to anonymize data when necessary. Permissions management, including maintaining up-to-date employee and user permissions, is equally important.
Integration between systems might pose an issue on the system end, especially if different providers are reluctant to share proprietary data. Organizations should be aware that sharing data with other providers is often in their best interests, leading to better outcomes and reduced costs for both parties.
Finally, using healthcare data algorithms raises the possibility of bias built into the system. At least one commonly used risk-scoring algorithm was found to have racial bias. In the long run, regulation will be required to ensure that AI algorithms are fair and responsibly deployed. Organizations and developers can do their part by ensuring that the AI algorithms they deploy for healthcare analytics are transparent, explainable and compliant with the Health Insurance Portability and Accountability Act (HIPAA) and other privacy guidelines.
Informing users about what data is being collected and enabling them to provide input on how it is used can increase trust in insurers and address ethical issues.
The future of health data analytics
A KPMG study in 2015 cited 10 percent of healthcare companies used advanced data and predictive analytics tools. That number has surely grown. Data analytics tools geared toward the healthcare industry are becoming more advanced and numerous. AI and ML will continue to be deployed to cut redundant processes, increase efficiency and perform failure checks.
The use of data analytics has become ubiquitous among health insurance providers. For businesses, data analytics is driving improvements in efficiency and cost reductions.
On the member end, analytics can provide users with tailored care, information and recommendations, reducing costs and improving patient outcomes. Real-time data analytics can expand the ability of insurance companies to provide customized service to members, as well as identify and reduce inefficiencies and the associated costs.
Healthcare insurance’s future involves incorporating AI and ML tools to make more accurate predictions, prevent failures and reduce costs. While the benefits are clear, using big data in healthcare inherently raises privacy and ethical concerns. Oversight, governance, and a strong focus on compliance and data security are necessary to ensure positive outcomes for insurance providers and members.
Surjeet Singh has more than 12 years of experience in the field of product development in a variety of industries including healthcare, insurance, and banking. He currently works in healthcare DevOps and manages multiple complex implementation projects, driving transformative IT initiatives. He can be reached at surjeetba@live.com.