Deciphering the AI spectrum: Advancing with precision and caution
From rudimentary algorithms to generative AI, organizations need to better manage the next transition in healthcare technology.
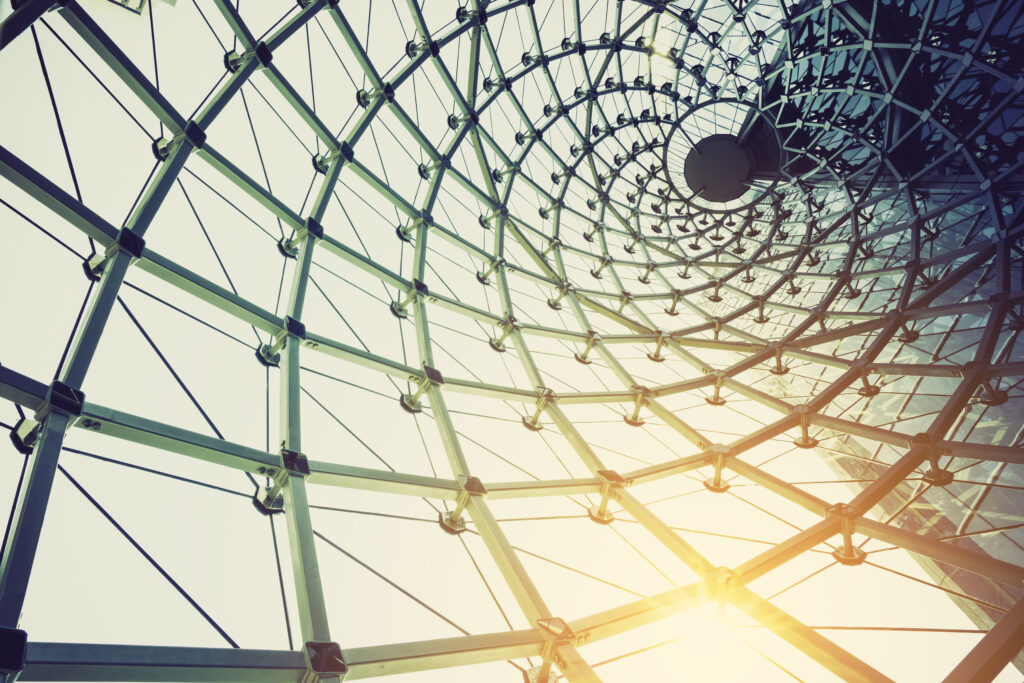
Interest in the adoption of artificial intelligence in healthcare has grown considerably over the past year. Google Trends data show a sharp increase in searches for “AI in healthcare.”
More than two-thirds (68 percent) of hospitals and health systems use artificial intelligence (AI) in some clinical or administrative capacity, according to a recent Becker’s Healthcare survey on AI adoption in healthcare.
While that sounds impressive, many healthcare organizations are working with vendors who use relatively rudimentary “AI” (things that are questionably AI) such as simple rules-based or statistical algorithms. It would be more interesting to conduct a survey of how many hospitals use more modern frameworks such as deep learning (DL) and large language models (LLM).
The noise and marketing hype surrounding AI has blurred important distinctions between various types of AI and their capabilities. This creates confusion in the market, which increases the temptation to lump everything that seems “AI-like” into one category. It’s no wonder that some providers find it difficult to trust vendor claims.
Rudimentary AI at work
Two primary examples of more rudimentary algorithms in use by provider organizations today are rules-based instructions and robotic process automation (RPA). In both cases, highly defined steps are baked into the processes for a program to work, and inevitably, the more rules-based and rigidly defined a process, the more brittle it is.
A good example of rules-based algorithms in clinical documentation would be setting up a process such that all cases within a specific set of diagnosis-related groups (DRGs) are automatically flagged for review based on past findings. These DRGs are “prioritized” for review based on statistical studies of historical behavior, such as where prior diagnosis opportunities have been found. Because that logic is hard-coded based on historical data, any changes – say new findings that impact the DRG like social determinants of health – invalidate those rules.
An example of RPA is programming a bot to navigate payer websites for various needs (for example, eligibility lookup). When the payer changes the user interface for their website, the bot breaks down, and new scripts must be written to adapt. And as the world is a constantly evolving place, organizations quickly find maintaining these bots to be labor-intensive, time-consuming, and costly.
How modern AI is different
In contrast, modern AI frameworks provide the ability to learn from data, as opposed to relying on set rules. This enables users to ask generalized questions, enabling complex decisions and considerations of context that are beyond the reach of simple statistical systems. A hallmark of these frameworks is the ability to adapt to changing conditions over time – such as learning from user feedback on whether they agree or disagree with a recommendation.
The flip side is that many of these models are nondeterministic – the same input does not always generate the same output. If you ask ChatGPT the same question twice, you may not get the same answer. The propensity of these AI systems to produce variable outputs – and possible hallucinations – means that hospitals or health systems that employ these must have a plan to monitor the quality and consistency of output.
As an example, modern models that are able to move beyond DRG prioritization and understand the entire clinical context around a patient’s hospitalization, including not just the values but the intent behind labs drawn, medications administered, and procedures ordered, but creating an explainable audit trail so that CDI and Coding professionals can monitor the accuracy of the output is key.
AI in healthcare, today and tomorrow
As hospitals learn how to deploy the AI of today, the AI of tomorrow is being built. As an example, neural networks can be used to detect valvular disease using electrocardiograms – something that even expert cardiologists are unable to discern. Foundational models like RETFound can detect Parkinson’s from scanning retinas.
And of course, generative AI (Gen AI) models such as ChatGPT, which are most responsible for public interest in AI over the past year, are being harnessed by healthcare organizations to enhance communications with patients, summarize patient histories for clinicians, automate administrative tasks and more. Gen AI has the ability to create new content – including text, images, and even code – based on information it has ingested.
Unfortunately, Gen AI models today have a troubling tendency to “hallucinate,” or invent facts, rendering them unsuitable in clinical settings. Until healthcare organizations can be certain they are working with clinically validated data, Gen AI’s use by providers, health systems and health plans should be limited and closely monitored. In part because of these hallucinations, 60 percent of Americans say they aren’t comfortable with providers using AI to diagnose their conditions and diseases and to recommend treatments. Introducing AI into administrative workflows can serve as a way for health systems to test the waters, realize tangible results and achieve buy-in from stakeholders.
Provider organizations must understand the capabilities and limitations of various AI technologies and models so they can choose the solution that best fits their specific needs and allows them to meet their goals. For hospitals and health systems evolving from rudimentary AI to more modern AI tools, it’s vital to implement monitoring processes to ensure quality control as their organizations migrate from deterministic algorithms to nondeterministic tools and outputs.
But it’s worth the effort: AI will introduce a new era in healthcare, and organizations that successfully deploy, learn from, and expand their use of AI will gain efficiencies, capture revenue, and improve care quality.
Michael Gao, MD, is CEO and co-founder of SmarterDx.