Why using AI in healthcare must balance innovation and patient trust
There are both promises and pitfalls in using generative AI in patient care, and providers need to begin work to resolve those issues now.
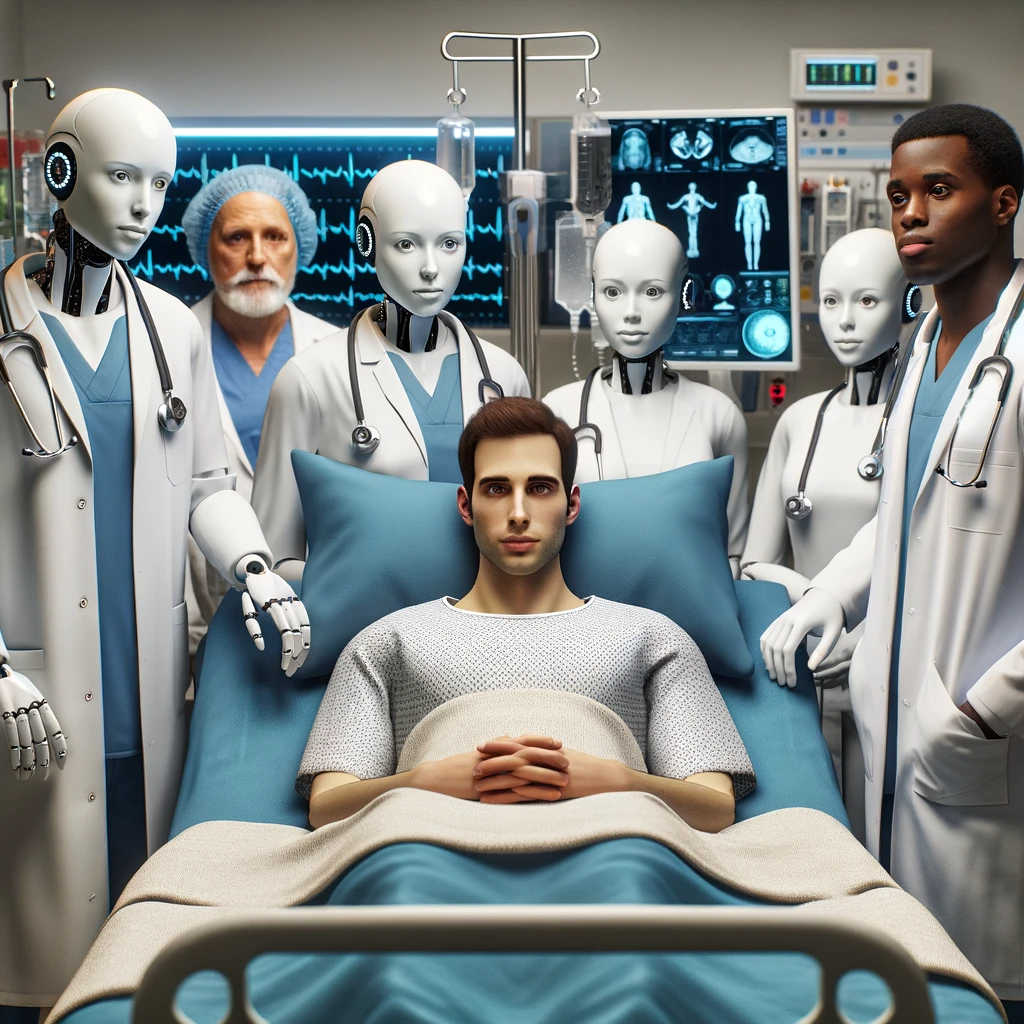
While artificial intelligence is not new to the healthcare industry, OpenAI’s recent release of GPT-4 and the introduction of other generative AI solutions has spurred a frenzy of activity around developing the next digital health breakthrough.
The healthcare industry has already deployed AI to transform many of its processes, from automating repetitive tasks to analyzing large datasets for disease diagnosis and even developing new drugs. Now developers are racing to integrate the latest GPT-4-like solutions into patient-facing applications.
The question is: are healthcare systems and patients really ready for generative AI?
The answer is far from clear. For example, a recent study from Pew Research found that more than 60 percent of Americans would be uncomfortable with a provider relying on AI in their own healthcare interactions, especially if the technology is used to diagnose a disease or recommend medical treatments. The study cited other patient concerns, such as racial bias, medical errors and health privacy as potentially dimming AI’s bright future.
If patients already feel like a number when they receive healthcare services, interacting with a chatbot is unlikely to change their sentiment. But when used responsibly, the technology has the potential to be another useful tool in a healthcare system’s arsenal, and the industry shouldn’t shy away from deploying it wisely.
AI and the patient experience
One area in which AI could make a difference is patient experience. Approximately 68 percent of patients believe healthcare providers need to improve their interactions with patients.
Studies have shown that personalized digital communications can play an important role in improving the patient’s experience and driving better health outcomes. AI could be used to augment digital communications in a way that leverages patient data and insights to provide recommendations around frequency of communications, channel preferences, and guiding healthcare providers on the best ways to interact with patients and motivate them to be more involved in their own healthcare. This would need to be handled with the utmost caution, because letting a machine generate uncontrolled communications with patients is risky.
While generative AI holds great promise for improving patient engagement, it is critical to balance its benefits with a prudent examination of its implications and challenges, particularly in the following areas.
Data privacy. A top concern is protecting healthcare data privacy. The effectiveness of AI requires extreme quantities of patient data, which experts believe may open the door to a host of cybersecurity vulnerabilities.
According to a 2021 study published in BMC Medical Ethics, the nature of the implementation of AI raises concerns about the “access, use and control of patient data” because it is often in private (third-party vendor) hands. The study also pointed to potential issues in confidently anonymizing data through AI-driven methods, increasing the risk of data breach or manipulation. This means it is critical that healthcare organizations ensure that technology vendors are adhering to the highest security standards to shield patient data from abuse, including informed patient consent to leverage their data.
Patient consent. As AI becomes more integrated into healthcare, providers must be fully transparent with their patients about what technology is being used, what data is collected and how it will be protected.
Algorithmic biases. An AI red flag lives in the technology’s potential to “deepen racial and economic inequities.” According to the American Civil Liberties Union, inherent biases in the data used to train AI language models have been found in numerous examples, resulting in gender and racial unfairness. While a lack of representation among engineering teams is somewhat to blame, it will be incumbent upon healthcare enterprises and vendors to monitor healthcare data for bias errors.
The Chilmark Research AI and Trust in Healthcare Report states that organizations should be wary of vendors offering a purely technological approach to bias mitigation. Health leaders should pay attention to the dimensionality of digital health data and take the necessary steps to attend to this aspect during the validation process. Chilmark researchers also detail the need for diverse data science teams and an organizational commitment to ensure responsible AI and health equity.
As healthcare ecosystems actively work to reverse disparities and drive health equity, these biases must be considered. Having high-quality data can help combat racial biases and ensure that AI models are robust and fair.
In sum, it’s clear that keeping the patient in focus will be key to succeeding with AI applications in healthcare. There is no question that AI will play an increasingly important role in the transformation in healthcare, but ensuring that patients feel seen and heard, and guiding them to optimal care must remain a top priority.
Building a foundation of trust with patients and gathering feedback is critical when leveraging any new technologies, and this will be especially crucial to AI’s adoption and success.
David Floyd is senior vice president of engineering for Upfront Healthcare.