Why integrated datasets effectively drive efficiency, cost reduction
The use of multiple forms of data helps take advantage of new strategies for payers and providers in the era of data-driven decision making.
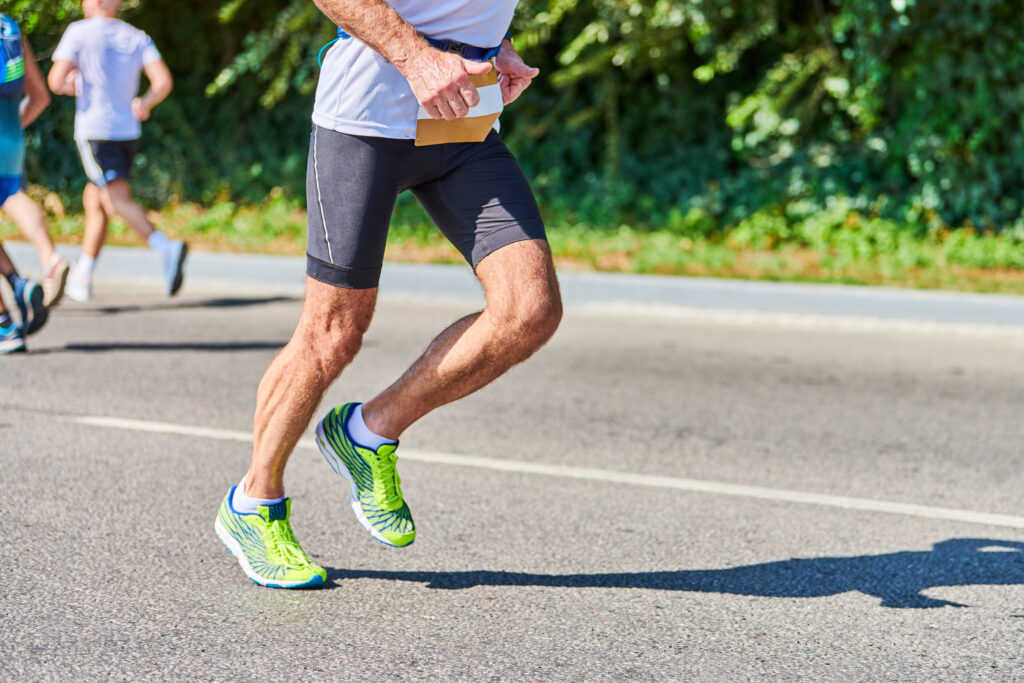
Annual Medicare spending is down according to a recent article in the New York Times and data from the Congressional Budget Office. Authors suggest multiple rationales for the improvement including the Affordable Care Act, value-based payment programs, greater focus on preventive medicine and the unfortunate loss of many high-risk Medicare members during COVID. Integrated data sets and the insights gleaned from them support healthcare’s achievement of these positive results.
Regardless of the reason, lower healthcare costs shine a glimmer of hope on all the hard work done by healthcare organizations in the past decade. Collectively, payers and providers have become much better at tapping data to understand problem areas and close operational gaps between each other to reduce healthcare costs. They’ve cut administrative waste and are working better together through more effective information sharing and data-driven change.
But progress is early. Much more work lies ahead.
For example, what more can be done to reduce healthcare costs using the vast treasure trove of data we’ve amassed and data that can be obtained from other sources? And how can we continually steer our organizations toward greater efficiency? Here are four new initiatives being implemented by both payers and providers to bend the cost curve using integrated data sets and new population health technology capabilities.
Focusing resource allocations
Data plays a significant role in the advancement of healthcare cost savings. Created by ubiquitous EHRs and amplified through interoperability, both payers and providers have access to larger amounts of data than ever before. This includes internally generated information, data exchanged between providers and payers, and supplemental data available from external sources.
With multiple and integrated data sets, organizations drill down deeper and identify new opportunities for cost savings at the individual level or across a specific population. Here’s one example.
Two patients may have the same diagnosis of congestive heart failure (CHF) with similar comorbidities and symptoms, according to EHR data. By layering an additional discrete data set for behavioral elements, systems can further flag which patient is most likely to make positive behavioral changes to improve health and which one won’t.
Knowing the difference between these two types of patients helps clinicians determine where to focus care coordination and interventional actions. The adage, “You can bring a horse to water, but you can’t make it drink,” applies here. Both CHF patients in this scenario are treated for the condition. But further investments in care coordination, preventive interventions, follow-up resources and more are most cost-effective for the “horse” that wants to drink — the patient that is willing to change behaviors to improve their condition and address comorbidities. The same intelligence is also being used by payers to manage members more cost-effectively.
The healthcare organization (whether the payer or provider) knows exactly where their resource allocations will be most fruitful. Resources are then spent where they are likely to have a positive impact on cost, quality and overall care outcomes. Drilling down on healthcare data empowers the organization to focus resources and reduce costs.
Drilling down on integrated data sets
The patient-specific example of drilling down on an integrated data set provides a glimpse of what is possible. The same type of intelligence applied at the population or community level magnifies an organization’s ability to allocate resources more efficiently.
For example, internal data may show that spending on a specific inpatient drug has increased in the past six months. Internal data identifies which patients are involved, their specific diseases and any patterns or trends in comorbidities. From there, another set of data can be applied to integrate socioeconomic status. For example, is there a subset of patients from the same ZIP Code that may have poor air quality or other environmental causes? Is income data available at the neighborhood level to further identify a patient cohort for free community resources or financial aid?
The goal of this and other data analysis is to specifically target the right set of patients that will benefit from proactive and preventive healthcare interventions — before they become costly inpatient admissions. New technology including artificial intelligence, machine learning and robotic process automation, advance the pace at which payers and providers can glean these insights, and broaden their reach across patient populations.
Ideally, payers and providers work collaboratively to shift focus from high-risk patients (those already being treated for disease) to risking-risk patients (those who are most likely to develop the disease).
Cost savings vs. cost avoidance
Justifying resource allocations to engage with rising-risk patients is another challenge to consider. We’ll never know with certainty if a rising-risk member will become an acute, costly inpatient. Common sense tells us costs rise when low-lying and preventable conditions become acute disease episodes. In a report conducted by The Lancet Public Health, more than a quarter of the U.S. healthcare spending was related to avoidable and preventative illnesses.
Some organizations may struggle with the cost savings vs. cost avoidance conversation. However, it is an important conversation to have across an organization to enable successful population health and preventive medicine programs over time.
The next step is to ensure best-practice patient and member engagement to ensure the optimal return on investment for proactive and preventive interventions.
It’s art, not science
According to Joe Babaian in The Healthcare Leadership Blog, the last mile of healthcare is the “link between you and the healthcare network touch point — where care is delivered.” Babaian further explains that this last mile can be a ride to the clinic or an upload of remote patient monitoring data into the provider organization’s EHR.
I define healthcare’s last mile as the ongoing member engagement or patient outreach that must occur to make a difference, improve care outcomes and reduce healthcare costs. This outreach isn’t typically conducted by physicians – it is the work of other ancillary clinical professionals, care coordinators, outpatient navigators and community health workers.
These professional teams seek to establish the same trusted, long-term relationships that patients have with their primary care providers. However, this trusted relationship must now be built over the phone, via text or by using other digital means instead of in-person physician visits. It is much harder to develop, but it is equally valuable to reduce costs and improve quality.
There are four essential qualities of a successful member and patient engagement professional.
They don’t stick to a script. They go beyond check-the-box medical screening conversations to build a trusted relationship with the patient or member.
They are great listeners. These professionals are adept at compassion and empathy. It’s their approach that matters.
They are prepared. They are fully armed with a ready arsenal of community resources to deliver immediate and helpful interventions.
They can discriminate. They know which horses are willing to drink at the trough and can convince them to take big swallows through follow-up care, community programs, exercise, diet and other preventive interactions.
Because payers and provider organizations lack sufficient staff to follow hundreds of patients every day, they often outsource outreach and follow-up to agencies that specialize in high-risk and rising-risk populations. Their expertise in communicating with patients or members is an art form — and a highly valuable strategy to achieve cost reductions in healthcare.
These teams are also uniquely qualified to ask the sensitive social determinants of health questions that primary care physicians lack the time or staff to capture. By enriching basic social determinants data with other outside sources of data and insights collected through member/patient engagement teams, full integration of data sets occurs.
Healthcare costs will continue to be reduced when organizations mine integrated data sets to uniquely identify members, patients and populations willing to modify their health behaviors. Armed with this knowledge, resources are properly allocated to assign the proper healthcare services, steer optimal preventative interventions and align community programs successfully.
Yousuf Ahmad is CEO of AssureCare.