Why AI-driven advances will revolutionize patient care in 2024
Generative AI’s power will be harnessed to transform medical imaging, combat radiologist burnout and better personalize cancer treatment.
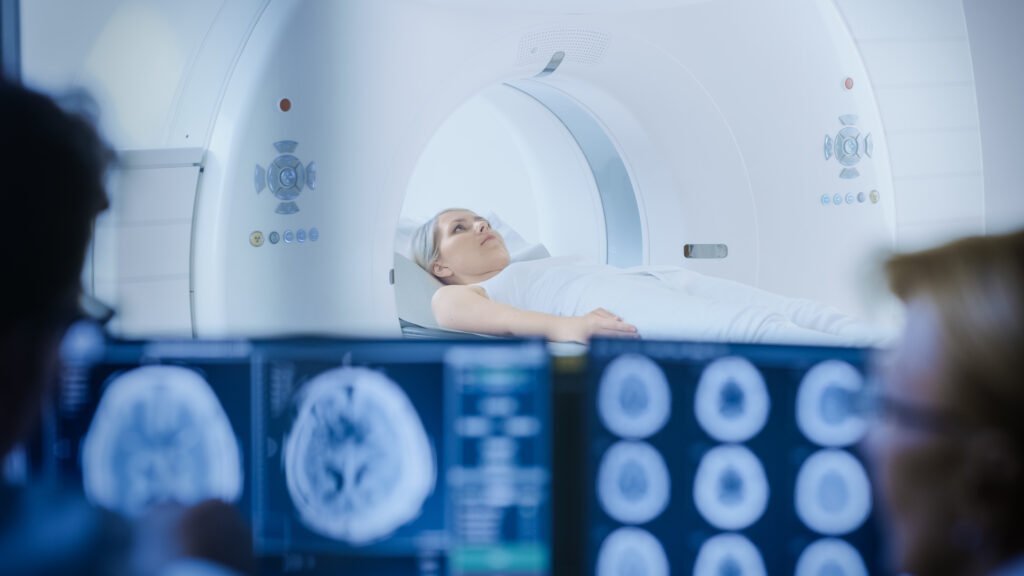
Generative artificial intelligence had its breakout moment in 2023 as the most advanced chatbot yet, ChatGPT, captured the public’s imagination by demonstrating the extraordinary power and versatility of generative AI.
As the public has already seen, generative AI (GenAI) has a remarkable ability to create new content – including images, music and text – that grows increasingly realistic over time because the technology actually learns as it goes. The core of GenAI is foundation models, which are changing the game by learning from a massive pool of data, giving them an almost human-like understanding of the world. This versatility means they can adapt to a variety of tasks. Foundation models are not just transforming technology; they're reshaping industries like education, healthcare and business, making our work more efficient and our lives easier.
In healthcare, generative AI could use complex algorithms to analyze large troves of patient datasets to create new “content” – everything from better medical images to actionable data-driven insights that improve provider efficiency and patient care.
The underlying technology powering generative AI is deep learning neural networks. These networks mimic the structure of the human brain by activating layers of connected nodes that process information. Just as different body parts work together harmoniously, deep learning integrates various facets of machine learning to create a comprehensive network of information.
This layered approach enables AI-powered technologies to build a detailed “snapshot” of vast volumes of data from which clinical insights can be derived. Deep learning can provide clinicians with more detailed and actionable information, empowering them with the insights to diagnose and care for patients with speed and efficiency across various anatomies.
Fears of AI replacing human clinical decision-making are largely overstated. Deep learning algorithms don’t make treatment decisions. Generative AI should be regarded as an “intelligent assistant” offering value-added ways to empower the provider to spend more quality time at the bedside and deliver better, more personalized care to patients.
While still in its nascent stages in the medical technology field, deep learning advances have begun to mirror the rapid trajectory of recent advancements in data storage and data aggregation.
What does deep learning hold for patients and providers? Here are three predictions for how generative AI will change healthcare.
Medical imaging will be revolutionized
Radiology is a crucial component of modern healthcare, enabling clinicians to visualize and diagnose a wide range of medical conditions. In recent years, the field has benefited from significant technological advancements that will revolutionize the way medical professionals diagnose and treat various illnesses.
Modern imaging tools now use deep learning for imaging reconstruction. One of the biggest problems in radiology that deep learning can help address is compromised MRI image quality because of challenges related to patient movement and excessive noise. Deep learning can enhance images in the face of these obstacles, reducing the need for repeat scans while enhancing the accuracy of reports so clinicians can make better diagnostic and treatment decisions.
In cardiac imaging, for example, deep learning has already paved the way for a 12-fold increase in image acquisition, enabling imaging within a single heartbeat. AI-powered deep learning can eliminate the need for repetitive breath holds, enabling patients with arrhythmias and difficulties holding their breath to benefit from cardiac MRIs.
In addition, generative AI could significantly benefit imaging in radiology by improving image quality, interpretation and workflow efficiency. It could assist in tasks like image reconstruction, denoising and artifact reduction, leading to clearer and more accurate diagnostic images. Additionally, generative AI models can aid in automating the analysis of medical images, helping radiologists by providing faster and more precise insights. This potentially could lead to earlier detection of abnormalities and improved patient outcomes.
Radiologist burnout will be reduced
The Association of American Medical Colleges (AAMC) projects a shortage of as many as 41,900 radiologists in the United States. The Royal College of Radiologists reports a 33 percent shortfall of radiologists in the United Kingdom, and that is estimated to increase to nearly 44 percent by 2025.
Many active radiologists are considering leaving the healthcare industry. A 2022 survey of 13,000 radiologists found that 49 percent reported burnout. The top cause of burnout (noted by 60 percent) was excessive bureaucratic tasks.
Using deep learning, we’re seeing a growing emphasis on driving efficiency and improved productivity across radiology departments. AI can continue demonstrating its effectiveness in radiology by automating repetitive tasks, monitoring quality during imaging acquisition, detecting abnormal patterns in intricate image data and aiding clinicians in diagnosing patients more quickly.
Initial use cases for generative AI are focused on creating operational efficiencies for bedside predictions, enhanced scheduling, streamlining electronic medical records and offering remote voice interface to help increase provider time with patients.
In the not-distant future, expect to see GenAI used throughout the imaging product lifecycle from image acquisition, image quality augmentation, patient monitoring and clinical decision support. These advances will address burnout by freeing up radiologists to spend less time on bureaucratic tasks and more time on patient care.
Personalized cancer care will improve
Piecing together a cancer patient’s entire medical history across their care pathway can lead to better clinical decisions. Machine learning and generative AI will be able to identify relevant data, anomalies and patterns in patient profiles.
AI not only aggregates data across electronic medical records, medical devices, genomics and treatment protocols, but it also could synthesize the data to support personalized treatment recommendations. This includes longitudinal multimodal data, including medical imaging, lab results and medical records. This will help clinicians make informed decisions at the right time, helping to ensure each patient receives the most appropriate care tailored to their specific needs.
Here’s an example: One of the greatest diagnostic challenges for pulmonary oncologists is determining the malignancy of a lung nodule – a suspicious lesion that may be benign or malignant. Incidentally detected pulmonary nodules present an indeterminate cancer risk and are incredibly challenging for clinicians to diagnose and manage, leading to delayed treatment for patients with cancer and invasive procedures for healthy people. The integration of imaging and medical device data with AI-enabled solutions through deep learning can help providers make a more accurate diagnosis and take the right action at the right time for each patient.
Overall, expectations for generative AI suggest that the integration of deep neural networks into medical technology will be a game-changing advancement that revolutionizes medical technology and equips overworked healthcare providers with accurate, data-driven insights to improve patient care.
Abu Mirza, MS, is the Head of Digital Engineering & Products at GE HealthCare.