How to better capitalize on AI by understanding the nuances
Artificial intelligence has promise, but organizations must understand its different types and be able to separate potential benefits from marketing hype.
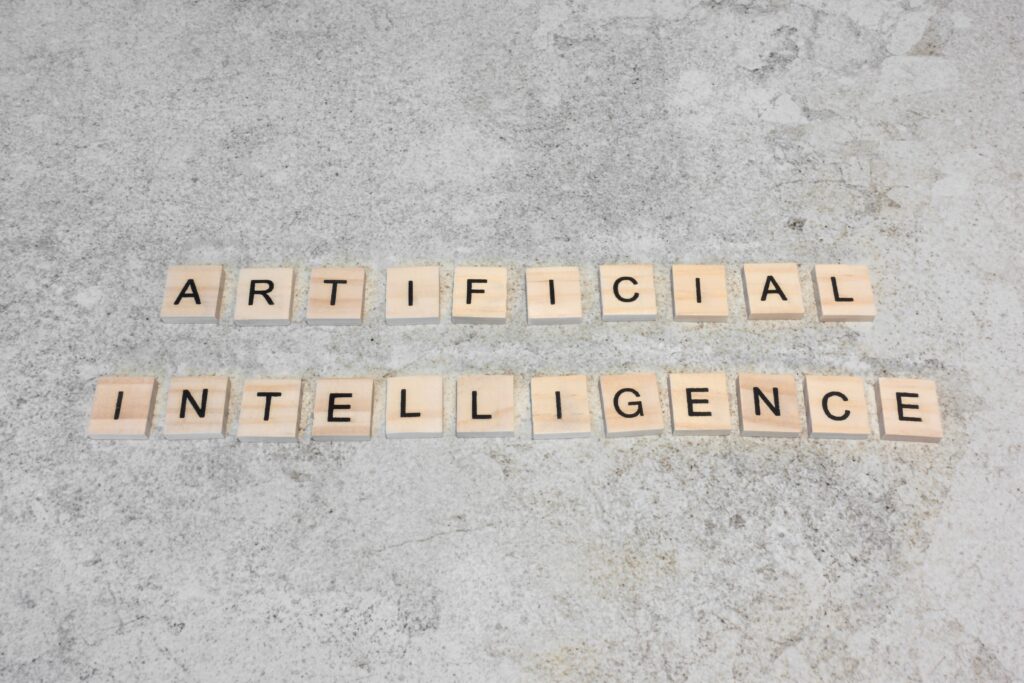
Artificial Intelligence holds immense promise in revolutionizing healthcare, but for many healthcare professionals, it remains a complex and enigmatic technology.
To use it wisely, it’s important to understand the intricacies of AI, provide insights into how healthcare institutions can develop effective AI strategies, assess AI's compatibility with existing workflows and technology platforms, and, most crucially, quantify the true return on investment (ROI) that AI can bring to healthcare.
Deciphering the AI enigma
It’s crucial to first grasp the essence of what AI truly is. At its core, AI refers to computer programs capable of performing tasks that typically require high-level mental processes, including perceptual learning, memory organization and critical reasoning.
AI was first coined in 1955 by John McCarthy, an MIT professor, as technology that could perform tasks that require human-level cognitive thought. However, AI is not a monolithic concept – it encompasses various approaches and types, each tailored to address specific challenges and scenarios.
Rules-based. This approach is sometimes sold as “AI,” but relies on predefined rules and logic. Prior iterations of robotic process automation often employed this.
Statistical inference. Statistical models and data analysis are used to make predictions based on historical data. These traditionally are used to generate correlations (for example, if a patient is on oxygen, then there is higher likelihood of acute respiratory failure) but can fail to account for confounding factors or alternative explanations (for example, patient is on home oxygen, not new oxygen).
Generative. Generative models create new content that mimics human creativity, such as generating art, music or text.
Natural language processing (NLP) and natural language understanding (NLU). NLP focuses on enabling machines to understand, interpret and respond to human language; there is no strictly defined difference between NLP and NLU.
Machine learning (ML) and deep learning (DL). These are subsets of AI that empower machines to learn from data and improve performance using neural networks and other advanced techniques.
Debunking AI myths
One of the primary misconceptions surrounding AI is the belief that a one-size-fits-all approach exists. In reality, the effectiveness of an AI approach — or a combination of approaches — depends on the specific problem it aims to solve. Healthcare institutions must carefully consider the nature of the issue at hand and tailor their AI strategy accordingly.
Furthermore, the term "AI" can be misleading. Not all companies that contend they are offering AI solutions employ the same technology or methodologies. It is essential to recognize that AI itself encompasses a multitude of methodologies, each yielding different results. Therefore, healthcare leaders must exercise due diligence in understanding the technology behind the label.
Additionally, building robust AI systems requires significant expertise and experience, and not all companies marketing AI possess the requisite capabilities. Unlike regulated food labels governed by the USDA, AI technology lacks standardized nomenclature, enabling companies to label their products as they see fit. This requires thorough scrutiny and evaluation of AI providers to ensure their technology aligns with the healthcare institution's goals and needs.
Moving beyond hype and haste
AI has immense potential in healthcare, but adopting this technology should not be rushed or driven by industry hype.
One of the critical areas where AI can make a substantial difference is in alleviating physician burnout. AI can streamline documentation processes, making them more efficient and reducing the administrative burden healthcare professionals now face. However, hastily rolling out large-scale AI initiatives can lead to regrets and difficulties in garnering buy-in or measuring outcomes.
For hospitals with narrow operating margins, embarking on AI implementation through broad strategies with vague outcome measurements is not advisable. Instead, a more prudent approach involves implementing solutions that impact only a handful of users, such as in the revenue cycle, enabling clear and meaningful ROI definition.
Healthcare institutions can further mitigate financial risks by structuring contracts with vendors on a contingency basis, diverging from traditional SaaS contracts.
Embracing AI with clarity and purpose
AI in healthcare has the potential to transform patient care and alleviate the burden on healthcare professionals. However, it requires a clear understanding of the technology, strategic planning and the ability to measure ROI effectively.
Healthcare leaders must actively engage in the conversation surrounding AI regulation and best practices, ensuring that AI becomes a valuable and trustworthy ally in the ever-evolving landscape of healthcare. By doing so, hospitals and health systems can unlock the true potential of AI and deliver enhanced care to patients while optimizing their operations.
Michael Gao, MD, is CEO and co-founder of SmarterDx.