How AI can support health equity: Strategies for ethical application
While challenges persist, organizations can harness artificial intelligence to address healthcare disparities and improve patient outcomes.
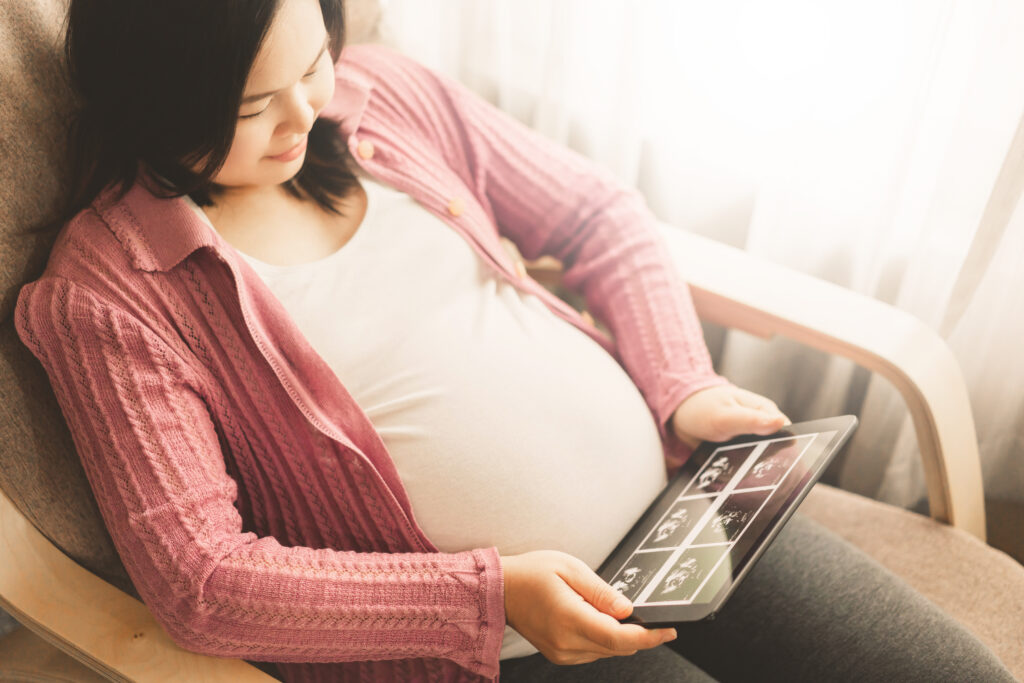
Health organizations, researchers and government entities are intensifying efforts to advance health equity. But accomplishing this worthwhile goal requires new strategies and technology solutions focused on removing barriers and reducing disparities throughout the entire healthcare system.
Artificial intelligence has emerged as a powerful tool to tackle this challenge head-on, offering immense potential to revolutionize diagnosis and treatment, accelerate operational efficiencies, and bridge gaps in both healthcare access and outcomes.
By using AI-driven predictive models and advanced analytics, organizations can identify underserved populations and take tangible steps to reduce systemic inequities. However, lacking a clear strategy and the necessary safeguards, AI-powered solutions can unintentionally exacerbate existing health disparities rather than solving them.
The risks are worth the rewards, but they still demand attention. As health organizations turn to AI and other advanced digital tools, decision-makers must take steps to ensure employees use tools ethically and effectively to make measurable progress in reducing health disparities.
Broadening access and outcomes
The most effective healthcare programs provide personalized care designed to foster trust, treat members as individuals and address patient needs, recognizing other factors such as social determinants of health (SDoH) in addition to medical conditions. It’s a lot to manage.
When applied correctly, AI can help organizations amplify and coordinate these complex efforts and better tailor services to historically underserved communities, especially at the scale needed to make a meaningful difference.
For example, AI solutions can offload the work of analyzing a member's personal data and health history. Based on these insights, AI tools can then outline a customized treatment plan, accounting for unique details, such as a person’s chronic health condition or a need for culturally tailored resources or education.
Likewise, if a patient has historically missed follow-up appointments because of a lack of transportation, AI-powered tools could not only help this person locate and book an appointment, but go one step further and arrange alternative transit options, such as a pick-up from their provider or a ride-hailing service.
AI can also play a role in enhancing prenatal and postpartum care by guiding expectant parents throughout their pregnancy journey, empowering members and equipping them with personalized resources and support via text and digital platforms. For example, through conversational AI and natural language understanding (NLU), health plans can connect pregnant members with the nearest clinics, transportation options and culturally competent educational content, such as streaming videos of people from a common background who share their own pregnancy journeys.
Comprehensive, accessible care like this is crucial because the U.S. holds the highest maternal mortality rate among developed nations. In fact, up to 60 percent of pregnancy-related deaths and adverse health outcomes could be prevented by expanding access, improving care and providing stronger education and resources. Such programs also contribute to reducing maternal health disparities and serving diverse communities.
This area is just one of many examples that demonstrate AI’s immense capacity to broaden healthcare access and improve health outcomes. However, there has also been general criticism about the use of AI in healthcare, and concerns about how biased algorithms might undermine health equity goals in the absence of appropriate precautions.
3 ways to reduce and remove AI bias
AI holds tremendous promise in helping organizations improve health equity. As AI-powered solutions are leveraged, here are three essential steps to mitigate potential biases and ensure AI tools support health equity goals.
View data sources with a critical eye. AI systems are only as good as the data that feeds them. In healthcare, biased data is particularly challenging in light of historical disparities and the vast data gaps among people of color and other marginalized communities found in research, academia and other institutions. Without measures to ensure data quality, accuracy and representation, AI tools will reflect bias in datasets and generate erroneous — and potentially harmful — conclusions.
As AI tools are adopted and integrated, organizations should actively work to address data gaps related to the treatment and experiences of marginalized communities. Start by setting high standards and adhering to industry best practices, such as those set by the Coalition for Health AI. At a minimum, this should include:
- • Establishing strong data quality, collection and curation practices.
- • Implementing algorithm governance that monitors AI outcomes.
- • Adopting pattern-matching capabilities.
- • Applying frequent data analysis and evidence-based assessments to evaluate the effectiveness of AI applications.
- • Conducting regular audits to identify problems and rectify biased outcomes.
Lean on human expertise. Despite rapid advancements in AI, humans still play a vital role in applying and managing AI tools. Technology investments should be paired with trained experts who can actively monitor AI outcomes to minimize potential biases, ensure alignment with ethical standards and see through insights generated.
Humans also bring intuition, empathy and personalized care to the table — essential qualities in healthcare settings. Consider healthcare communication: While AI can expedite certain processes and produce mass volumes of messages, human input is still needed to provide cultural competency, nuance, sensitivity and a personal touch.
Moreover, manual expertise and oversight ensure even the most sophisticated technologies retain a human-centric perspective centered around patient needs. Human oversight applies the “reasonableness” test to AI recommendations and ensures the elimination of extreme outliers. In particular, humans play an important role in recognizing how personal experiences, Social Determinants of Health (SDoH), and other factors impact people's health and the development of individualized solutions.
Diversify your team. Diversity in AI development teams is not only an ethical imperative; it’s a strategic necessity. Diverse perspectives will significantly enhance the relevance and effectiveness of your AI applications and ensure all people and communities are considered in the development and deployment of AI-driven solutions.
Multi-disciplinary teams that include healthcare analysts, health economists, statisticians as well and data, ML and AI engineers can enhance the effectiveness of models, identify and solve problems more completely, and correct for data and algorithm bias more effectively. Adding frontline healthcare workers to provide first-hand observations as feedback further helps to gauge the validity of the AI models.
Diversity extends beyond internal technology teams to include senior leadership and member advisory committees who can provide valuable insights and lived experiences to inform and improve health equity initiatives.
Leveraging AI as a tool for good
As health organizations work to tailor services and improve health equity, AI technology shouldn’t be viewed as a catch-all solution, nor should it be viewed as an inherent problem. As with any task worth doing, the journey toward responsible AI simply requires a steadfast commitment to improving health equity — and understanding how digital tools can be leveraged for the benefit of all people.
By combining the best of AI and human expertise, organizations can take a more thoughtful and personalized approach to caring for members. This balance turns a potentially harmful technology evolution into a once-in-a-lifetime opportunity to help build a healthier, more equitable future.
Sanjeev Sawai is chief product and technology officer for mPulse.