Harnessing AI for clinical data registries: A guided approach
To be effective in using advanced technologies strategically, it’s key to define appropriate use cases for AI within data registries.
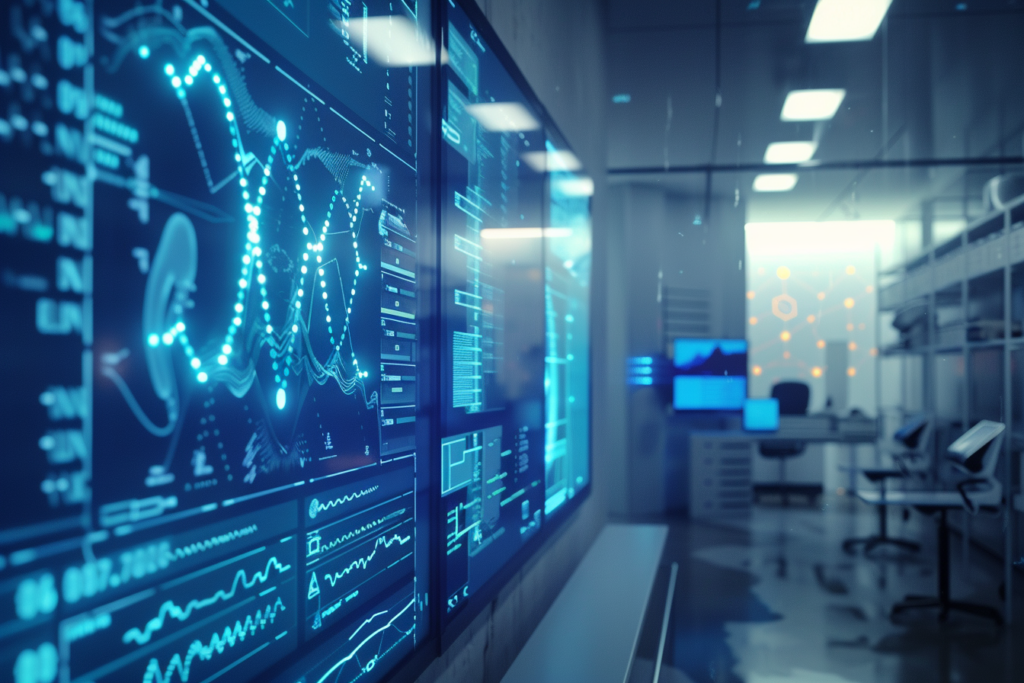
Amid a flurry of activity in the advanced analytics space – including OpenAI’s rollout of customizable versions of ChatGPT, the launch of Microsoft’s AI-powered Microsoft Fabric and Humane’s recent release of the AI Pin – industries are looking for ways to integrate natural language processing (NLP), artificial intelligence (AI) and machine learning (ML) into their business operations.
Healthcare is no exception. With the average hospital producing approximately 137 terabytes of data every day, stakeholders across the healthcare ecosystem are interested in using tools like NLP, AI and ML to streamline the extraction and analysis of this data. Potential use cases include developing clinical decision support tools that can improve patient care, augmenting clinical trial platforms to streamline trial operations and enhance trial diversity, and automating clinical workflows to reduce administrative burden and mitigate physician burnout.
While NLP, AI and ML (a collection of tools that will be generally referred to as AI in this piece) have the potential to transform and improve healthcare, their use does present certain risks. President Biden’s recent Executive Order on the use of AI notes that “irresponsible use could exacerbate societal harms such as fraud, discrimination, bias, and disinformation; displace and disempower workers; stifle competition; and pose risks to national security.”
In a similar vein, the World Health Organization recently published guidance for regulating the use of AI in healthcare. As such, businesses in heavily regulated industries like healthcare must tread cautiously with their use of AI.
Based on our substantial experience in leveraging AI, we see potential to derive value from healthcare datasets across the industry. This includes collaborating with various medical societies to build next-generation registries integrating AI. Additionally, AI applications can help streamline healthcare operations, such as reducing waiting lists in hospital settings.
Appropriate use of AI in clinical registries
In light of the variety of use cases for AI across the healthcare ecosystem, it is no surprise that many clinical data registry vendors are looking to integrate AI-based tools into their registry operations.
Clinical data registries often rely on a diverse array of clinical and billing data sources, leading to high variability in data formats and challenges with extracting useful insights from a large, diverse dataset. Integrating AI can help address these challenges, enhancing the value of the registry and reducing the amount of manual effort to maintain it.
AI can be used to identify, extract, encode and analyze data, ultimately helping registry operators to unlock the value of their datasets – but only if used appropriately. AI is best used for identifying opportunities to improve care quality, supporting research and de-identifying datasets – but AI should be avoided for calculating outputs that will be submitted to regulatory authorities.
Using AI to improve care quality
Clinical data registries often contain large volumes of unstructured data, such as free-text notes (such as patient charts or plans of care). AI is extremely valuable for extracting, analyzing and deriving insights from this unstructured data, which can help practices drive targeted quality improvement initiatives.
For example, NLP could be used to parse plan of care data for patients discharged from acute care facilities to identify how many opioid poisoning or overdose patients were prescribed naloxone at discharge. If within a particular practice, a large proportion of records indicated that opioid poisoning or overdose patients were not prescribed naloxone, practice leadership could implement a quality improvement initiative to educate providers about the importance of naloxone prescriptions in preventing future opioid overdoses, which are responsible for 75.4 percent of all drug overdose deaths.
AI can also be applied to derive insights from structured data elements. For instance, one study used ML on clinical registry data to identify variations in clinical decision-making between hospitals regarding the use of thrombolysis for stroke patients. These insights could be used to drive consistency in clinical decision-making across practices to improve health outcomes for stroke patients.
When used appropriately, AI can substantially reduce the time and effort required to gather insights from large volumes of healthcare data, helping practices to identify areas of opportunity and improve patient outcomes.
Using AI to support research
Clinical data registries provide access to a unique ecosystem of healthcare data that can be used to support quality improvement, clinical and cohort-based research initiatives. This research is key for assessing health outcomes, defining clinical best practices, and driving the development of novel treatments and procedures across specialties. AI can be used to extract and analyze large datasets to support a variety of research initiatives.
Registry data could be used to train ML models to support risk stratification of patient populations. For instance, one study developed an ML-based model to predict mortality rates among patients undergoing transcatheter mitral valve repair. As another example, NLP and ML can be used to extract and analyze data from free-text patient notes for a population of cancer patients to identify disease trajectories and predict adverse events.
AI can support a diverse array of research use cases, helping to inform clinical decision-making and support treatment innovation. Our previous collaborations with medical societies have demonstrated that research is a key value driver for academic medical centers and can enhance registry participation among large AMCs.
Using AI to de-identify datasets
Life sciences companies – including pharma and biotech organizations – may be interested in leveraging registry datasets for a variety of use cases, including monitoring adverse events, streamlining clinical trial design and recruitment, identifying label extensions, and informing drug pricing.
Using the data for these purposes requires de-identification – a process that can be accelerated by using AI. For example, NLP could be used to parse a registry dataset and remove all patient names, addresses and other personal identifiers so the dataset could be licensed to pharma companies to inform their clinical trial design.
Natural language processing provides a valuable and scalable method for de-identifying large datasets, with one study even showing that NLP systems performed better than human annotators in identifying and removing protected health information (PHI) from clinical notes. NLP can be used for parsing data from a variety of report formats, as one study found by using a natural-language-processing-based named entity recognition (NER) model to de-identify a dataset of seven different types of radiology reports.
Using AI for de-identification does carry a significant risk – improper de-identification can result in HIPAA breaches that carry significant financial penalties and violate patient trust. However, advisory organizations can be used to audit and confirm the integrity of de-identification algorithms, mitigating the risk of potential HIPAA breaches.
If risks are properly managed, AI can provide an effective, scalable way to mask PHI and enable the use of registry data for a variety of life sciences use cases.
Limitations in using AI
Qualified clinical data registries (QCDRs) are a special type of registry approved by the Centers for Medicare & Medicaid Services (CMS) for use in reporting to CMS as part of the Merit-based Incentive Payment System (MIPS). These registries can provide value to their users by streamlining the process of quality reporting.
Some of the MIPS measures rely on data elements that might be found in the patient notes. For example, one MIPS measure involves checking whether melanoma patients were entered into a recall system that includes a target date for the patient’s next exam and a process for follow-up with patients who fail to make an appointment.
While some of the data elements required for this measure can be found in standardized code sets – for example, diagnosis codes for melanoma – confirmation that patient data was entered into a recall system is typically found in free-text notes rather than a structured dataset. In this case, NLP could ostensibly parse the free-text notes for patients with a melanoma diagnosis code and identify what proportion of records met this measure.
However, using AI to extract and encode data from patient notes for the purpose of calculating MIPS measures may lead to false positives or false negatives because they are ultimately probabilistic in nature, resulting in incorrect MIPS scores. In the event of a CMS audit, QCDR operators must have adequate documentation to explain how the registry software calculated a particular score.
If NLP models were used in the calculation, it could create substantial audit risk because of challenges with proving how the NLP model came up with a particular result. This is especially true if the registry operator lacks access to the training data used to build the underlying model, which is often the case with mainstream AI applications. As such, we advise against using AI to calculate outputs that will be submitted to regulatory authorities and could be subject to audit.
Other considerations for using AI
Even when used appropriately, there are other risks to keep in mind when using AI.
For example, AI can provide inaccurate outputs in the form of hallucinations, which are particularly concerning when AI is being used to inform healthcare decisions. Technology vendors can mitigate the risk of hallucinations by regularizing the model, using sufficient and relevant data to train the model, and providing feedback to the model.
Another important risk is that using AI to inform clinical care may foster patient distrust, with one poll finding that three out of four U.S. patients distrust the use of AI in healthcare settings. However, based on the same poll, two out of three respondents indicated that they would be more comfortable if they had an explanation of how their healthcare provider is using AI, suggesting that transparency is key to maintaining patient trust.
It's clear that AI is a valuable tool for healthcare companies – including clinical data registry operators – and provides the potential to accelerate and improve the extraction and analysis of health data and enable a variety of use cases, such as improving care quality, supporting clinical research, and accelerating drug development. However, AI can also present substantial risks around patient trust and regulatory audits. As such, it is critical for healthcare organizations to ensure that they – and their technical vendors – use AI appropriately.
Nilesh Chandra is a healthcare expert and partner at PA Consulting leading the firm’s work on healthcare data platforms.
Steve Carnall is a healthcare data integration expert at PA Consulting and works with healthcare associations across specialties to build custom, scalable registry solutions.
Erik Moen is a health and life sciences expert at PA Consulting and supports clients across the healthcare ecosystem with digital- and data-related initiatives. Nori Horvitz is a healthcare data expert at PA Consulting and brings end-to-end experience in building healthcare registries.