Guardrails for progress: Governance as the unsung hero of innovation
Healthcare leaders know success with technology depends on governance that defines responsibility, enforces standards and aligns culture.
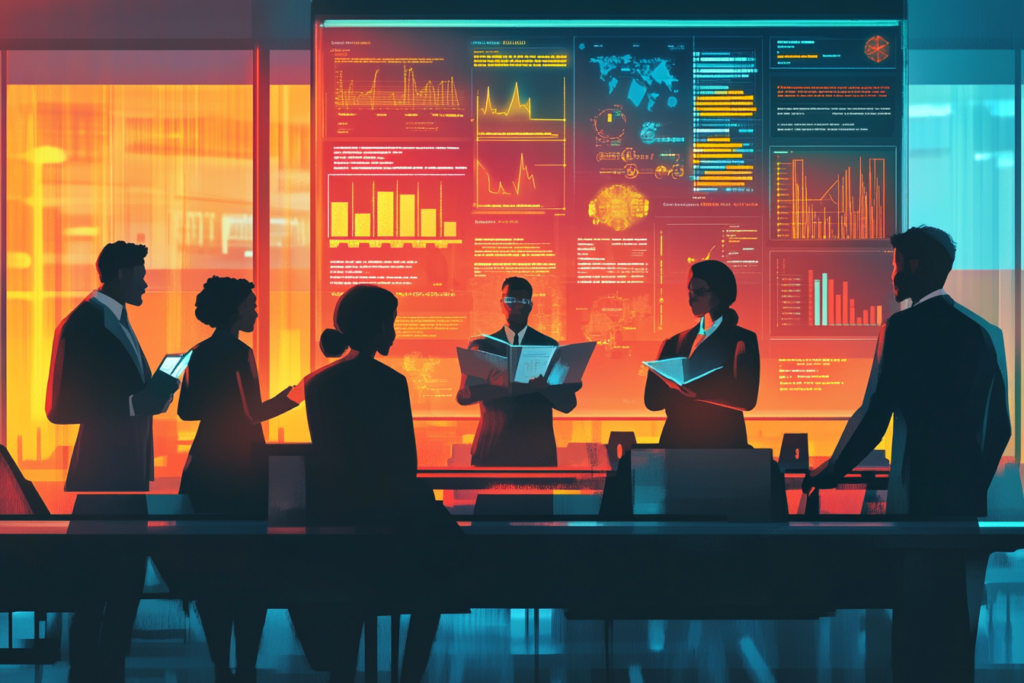
As the healthcare industry continues its transition to digital transformation, artificial intelligence is rapidly becoming an embedded tool in everything from clinical decision support to revenue cycle management.
But for all its promise, AI also gives rise to difficult questions about bias, trust and the ethical use of patient data.
Increasingly, healthcare leaders are acknowledging that technology alone doesn’t determine success. Instead, it’s the invisible infrastructure of governance — how organizations define responsibility, enforce standards and align culture — that ultimately determines whether digital innovation actually improves care.
“We must ensure that the data feeding our AI systems is accurate and unbiased to achieve reliable outcomes,” says Liz Griffith, a Fellow of the American College of Health Data Management and director of EHR education at uPerform. “Garbage in, garbage out isn’t just a phrase — it’s a root cause of failure if governance is an afterthought.”
A clear signal from the top
Federal leadership is sending a strong message. In late 2023, President Biden issued an executive order on the safe and secure use of artificial intelligence, warning that irresponsible deployment of AI could “exacerbate societal harms like discrimination and bias.”
The executive order emphasized the need for a “society-wide effort” that brings together government agencies, healthcare organizations and technology companies to establish clear ethical boundaries.
This echoes guidance released by the World Health Organization, which outlined more than 40 recommendations for ethical AI use in public health settings. At the center of both messages is the overarching truth that AI must be governed, not just engineered.
“Developing AI-driven risk stratification models requires a keen focus on interoperability, bias mitigation and adherence to policy frameworks,” says Julia Rehman, a healthcare analytics leader and ACHDM Fellow. “The technology is moving fast, and we’re building decision engines that need to be explainable, transparent and auditable.”
A ground-level view of responsible change
Rehman’s insights come from real-world experience. She recently led a cross-functional transformation initiative focused on analytics and enterprise data strategy.
“We aligned stakeholders, implemented governance structures and built change management protocols to ensure the systems we were standing up were not only innovative, but understood and embraced,” she explains.
At the team level, Rehman has made it a priority to embed these principles into daily operations. “To ensure teams remain adaptable, I’ve integrated ethical and compliance training into our core upskilling programs,” she adds. “It’s not enough to know how to run a model; you have to understand what it means when that model fails.”
Griffith concurs that education is just as important as technical performance. “While it may seem counterintuitive, the investment in people — training, onboarding, real-world scenario testing — is just as critical as choosing the right tools,” she says. “Without it, data governance gets abstract, and that’s when mistakes happen.”
Balancing transparency with innovation
In an era of predictive analytics, federated learning and large language models, the governance challenge is growing more complex. However, solutions are emerging.
A recent Health Data Management report spotlights health data utilities as one model for balancing interoperability with privacy. By coordinating data from clinical, social and behavioral sources while ensuring strong consent management and policy alignment health data utilities offer a way forward that integrates care delivery and ethical oversight.
At UC San Diego Health, Karandeep Singh, MD, its chief health AI officer, has emerged as one of the voices calling for deeper governance discipline. “Technology is rarely the only problem — usually it's a mix of process, social issues and navigating how things are currently done and trying to change those,” Singh told Health Data Management.
He emphasizes that building AI into healthcare is as much about changing systems and culture as it is about rolling out code.
Ethics is infrastructure
For organizations rolling out AI, the temptation is to go fast — going from piloting a solution to reporting results to expanding implementation. But without clear standards and human-centered values embedded in those efforts, there’s a huge risk that velocity will beget volatility.
That’s why governance must shift from being seen as red tape to being embraced as being as foundational as infrastructure. It’s not just a matter of compliance; it’s a foundation for trust.
“Technology doesn’t transform systems, people do,” Rehman says. “And people will only trust systems that reflect the values of the organizations they’re part of.”
Griffith puts it even more bluntly: “Governance isn’t about slowing things down. It’s about not blowing things up.”
As healthcare charges ahead into an AI-powered future, data governance is no longer a behind-the-scenes function; it’s a strategic necessity. The Fellows of the American College of Health Data Management are making it clear that strong governance is what enables innovation to scale, bias to be checked and trust to take root.
Employing best practices
Here are a few best practices these leaders recommend for organizations navigating the next wave of digital transformation.
Build explainability into every model. “We’re building decision engines that need to be explainable, transparent, and auditable,” says Rehman. AI tools must be understandable not only to data scientists, but to clinicians, regulators and patients.
Embed compliance and bias training into workforce education. Rehman emphasizes that training isn’t just about technical tools — it must include ethical literacy. “It’s not enough to know how to run a model; you have to understand what it means when that model fails.”
Treat governance as infrastructure, not overhead. Griffith cautions that sidelining governance will cost more in the long run. “Garbage in, garbage out isn’t just a phrase. It’s a root cause of failure if governance is an afterthought.”
Invest in real-world scenario testing and onboarding. According to Griffith, governance comes alive through application. “The investment in people — training, onboarding, real-world scenario testing — is just as critical as choosing the right tools.”