Gen-AI and Narrow-AI bridging technology in health and care
Healthcare organizations increasingly can leverage Generative and Narrow AI to support increased healthcare efficiency and better patient outcomes.
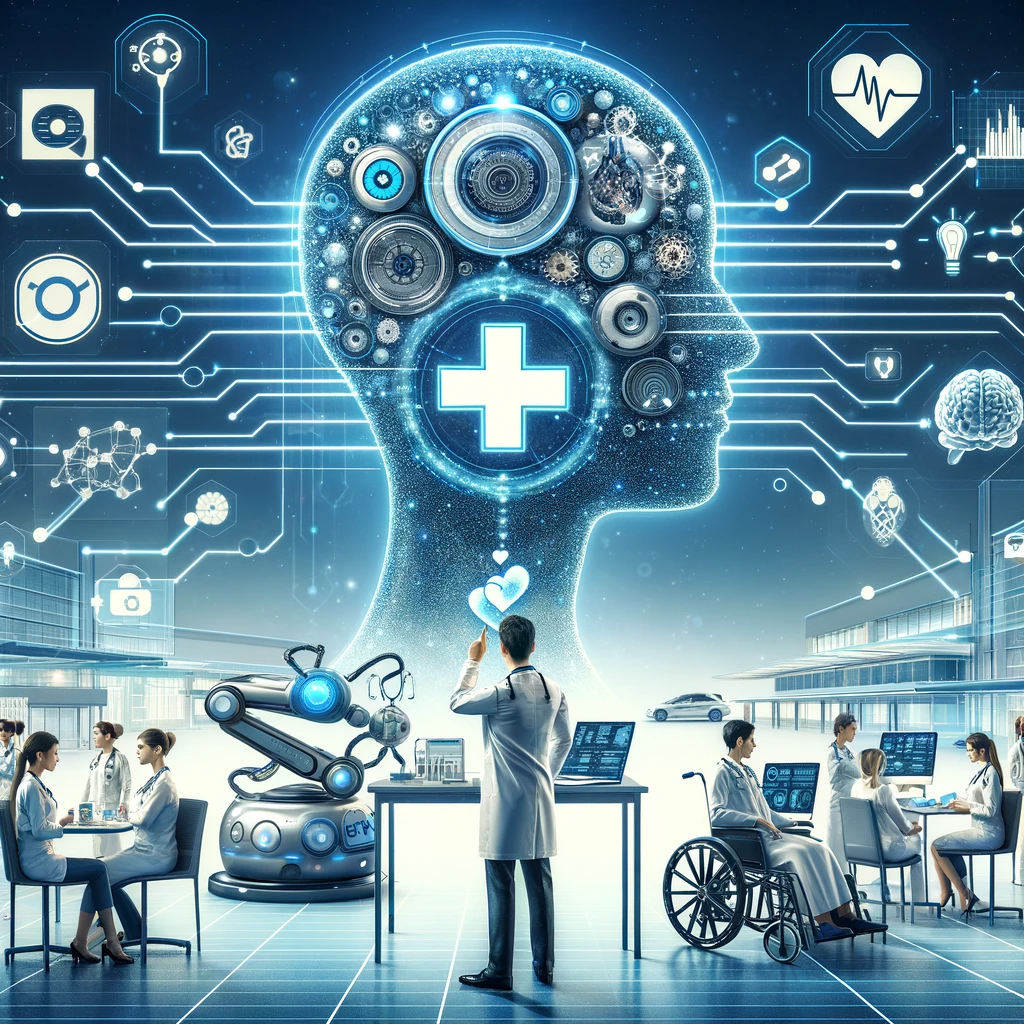
Healthcare organizations have tapped some artificial intelligence capabilities for several years. Predictive analytics, machine learning, image recognition, natural language processing — all of these are versions of AI, sometimes referred to as “narrow” AI.
Generative AI (gen-AI) tools that can create content on the fly, such as ChatGPT and BARD, are driving fresh excitement. Looking beyond that hype, what makes the value proposition of AI especially compelling now is how all these tools can work together to bring powerful new capabilities to healthcare organizations. Gen-AI plus narrow AI can reduce costs, lighten administrative burdens, enable greater personalization of care and, ultimately, help achieve better health outcomes.
Here are just a few of the key areas in which practical AI will reshape the healthcare industry over the next few years.
Streamlining claims and reimbursements
AI can enable faster and more accurate processing and reimbursement for healthcare organizations, as well as members and patients. Gen-AI engines digest data about contracts, claims, benefits, medical guidelines and standard operating procedures. Then, gen-AI can work alongside claims administration and medical records systems, augmenting the abilities of claims adjudicators and revenue cycle managers. Gen-AI can spot missing or inaccurate information in claims before they are submitted.
On the payer side, gen-AI can help adjudicators prevent inaccurate denials, automatically generate requests for specific documentation and other potential issues.
Gen-AI can also enable smarter pre-registration and registration procedures, helping to ensure the right eligibility information is captured upfront to streamline downstream claims processing. In addition, AI tools can enable patients and health plan members to receive more comprehensive, accurate information about their financial responsibility. AI-based solutions can even help suggest payment options tailored to an individual’s projected ability to pay.
Personalizing patient and member experiences
Gen-AI can greatly speed responses to questions from all healthcare stakeholders, whether about eligibility, claims status, prior authorizations or other concerns. With the right training, gen-AI models can recognize keywords and match them to their clinical synonyms and either provide responses or prompt human agents to handle the inquiry.
Working in real time, AI can provide feedback and relevant information. The feedback could take the form of customizing health risk assessment or social determinants of health questions as a member or patient is taking the survey. AI can prompt clinicians, care managers and service representatives about the next best action to take. Gen-AI also can review and summarize the content of 100 percent of health records, survey responses, contact center interactions and more, providing a wealth of data to use in training and continuous improvement programs.
Improving health outcomes
Gen-AI can find patterns in huge volumes of data as well as in one person’s data. With the right training and access to clinical data, gen-AI can help create individualized care management plans. It can enrich interactions with patients and members by producing personalized education materials and evidence-based care plans tailored to an individual’s demographic, social determinant of health and clinical data. Care managers would review the customized care plan and fine-tune it as necessary.
Care tailored to an individual’s history and needs should be more effective than generic approaches. Personalized health coaching to improve adherence can also be powered by gen-AI engines.
AI: Great work in progress
Ensuring the safe use of AI in healthcare has already triggered a federal executive order calling for regulatory guardrails. Healthcare organizations should understand differences among gen-AI models and their safety issues.
Popular public large language models (LLM), such as ChatGPT, “learn” from all the content available on the Internet. That vast public data pool contains inaccurate information and biases that may influence gen-AI created content and recommendations. These models also sometimes “hallucinate,” returning incorrect answers. Keeping humans in the loop to monitor and assess gen-AI results is critical.
Google, Microsoft and a variety of start-up companies are creating healthcare-specific LLMs for training gen-AI, which should help improve gen-AI clinical accuracy and results. Healthcare organizations may integrate these industry-specific models into their enterprise data environments. Gen-AI models often must be coupled with other machine learning algorithms, platforms, apps and analytical tools to deliver game-changing results. Bringing a healthcare-specific gen-AI model to the enterprise is more secure and private than sharing enterprise data with centrally managed, cloud-hosted gen-AI models.
Healthcare organizations that experiment with AI proofs of concept and pilot programs will gain a first-hand understanding of how gen-AI and narrow AI can improve administrative and clinical operations. That knowledge will be a solid foundation for increasingly sophisticated applications and advantages from AI, from minimizing tiresome administrative tasks to augmenting clinical expertise to improving healthcare experiences and outcomes.
Deepan Vashi is executive vice president and global leader at Firstsource.