Taking aim at health equity requires data, timeliness and precision
While some measures are being used, the country is not there yet. More accurate health indices require a more expansive approach.
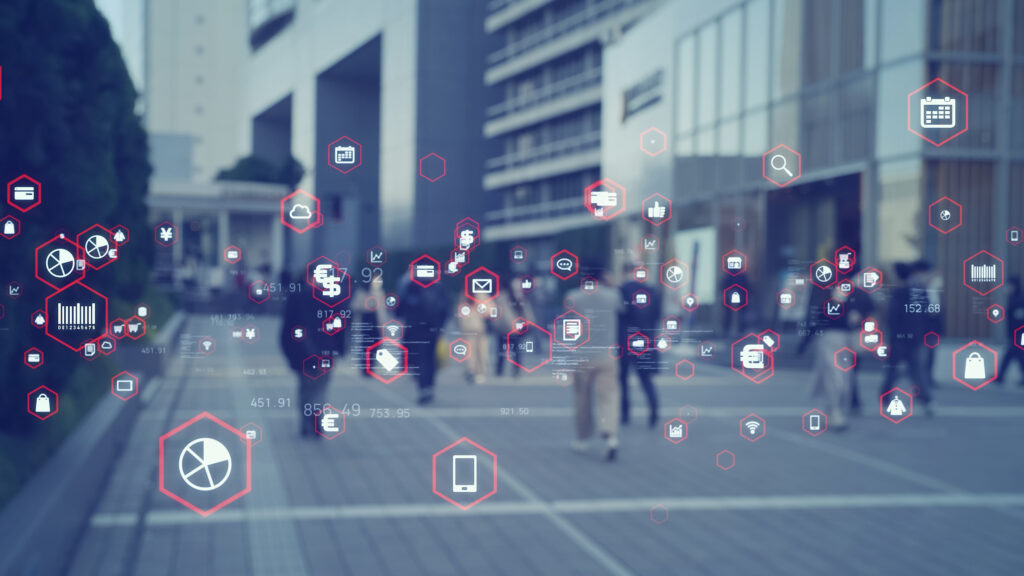
As the nation continues to reckon with health inequity, access to data increasingly enables organizations to pinpoint its causes and better target solutions.
Health inequities are correlated with many social factors that are identifiable as data points, such as poverty, crowded housing, air pollution, food deserts and access to transportation.
There are several indices created by various agencies and organizations that are working to quantify health equity, with private and public entities relying on these indices to direct resources. But not all indices are created equal, and where patient care is concerned – not to mention public funding – a close examination of these indices is worthwhile.
The problem is that these models often miss the mark because they are too broad, applying a one-size-fits-all approach to a country that is anything but homogenous. Take for example, the CMS payment model, in which physicians are paid more money to treat underserved populations, that may inadvertently be penalizing low-income people and communities of color in large, urban populations.
The model relies on a metric from the Health Resources and Services Administration’s Area Deprivation Index (ADI) to calibrate neighborhood social needs based in part on home values. Unfortunately, this metric can create distorted results in areas that have both very high- and very low-income households. This is something that holds true in many cities.
A more expansive index is needed to fully quantify and prioritize the inequities that communities are experiencing. The ADI leverages 17 social factors in six categories — income and wealth, employment, education, housing, transportation, and social environment — but with particular weight given to income and housing prices. Unfortunately, a lot of people suffering from health inequity are left out of that equation.
Improving accuracy
As a data scientist who has been working in this space for more than a decade, I can tell you that the discrepancy can be accounted for with a more robust set of data and using the right algorithm. This data is publicly available — it just needs to be normalized, quantified and timely.
When an index includes more data — health systems (percent uninsured, provider shortages, distance to a hospital), physical environment (air pollution, water pollution, hazardous waste and spill risk) and public safety (gun violence and crime statistics) — it produces a more accurate correlation. In the case of life expectancy, using the nine categories across 43 data points, again, all publicly available, yields a 75 percent correlation between social factors and life expectancy, compared with the ADI’s 40 percent correlation.
Another key to the accuracy of an index is the recognition that the U.S. is a large and diverse country, with different social needs in different places. One algorithm applied across the country will not account for the variability in the impact of different drivers of health in different communities.
Allowing the model to flex provides not only a strong correlation to life expectancy in all of the extremes of urban and rural spaces, but also provides extra information about how those models reflect the different importance of each social need in each community. High housing costs can reflect a wealthy population in some communities, while being a risk factor for housing insecurity in others.
Data at a local level
Knowing exactly what issues are affecting residents gives health systems perspective on the resources or partnerships necessary to better support their patient population. The more robust and timelier the data, applied at the most local level, the better the visibility for providers to fully understand their communities and the people who live in them — and to take a more precise aim at the causes that lead to health inequities.
Because a large portion of hospital employees live in the same vulnerable communities as a hospital’s patients, health systems have an opportunity through the right index to solve problems keeping employees from work (think lack of transportation, access to care). It would be inaccurate to say the possibilities are endless, but they’re darn near close.
Beth Godsey, senior vice president, data science and methodology, oversees analytical modeling, metric development and the hospital ranking/scoring methodology and framework at Vizient.